Transform Raw Data into Strategic Assets: The Future of AI-Driven Business Analysis
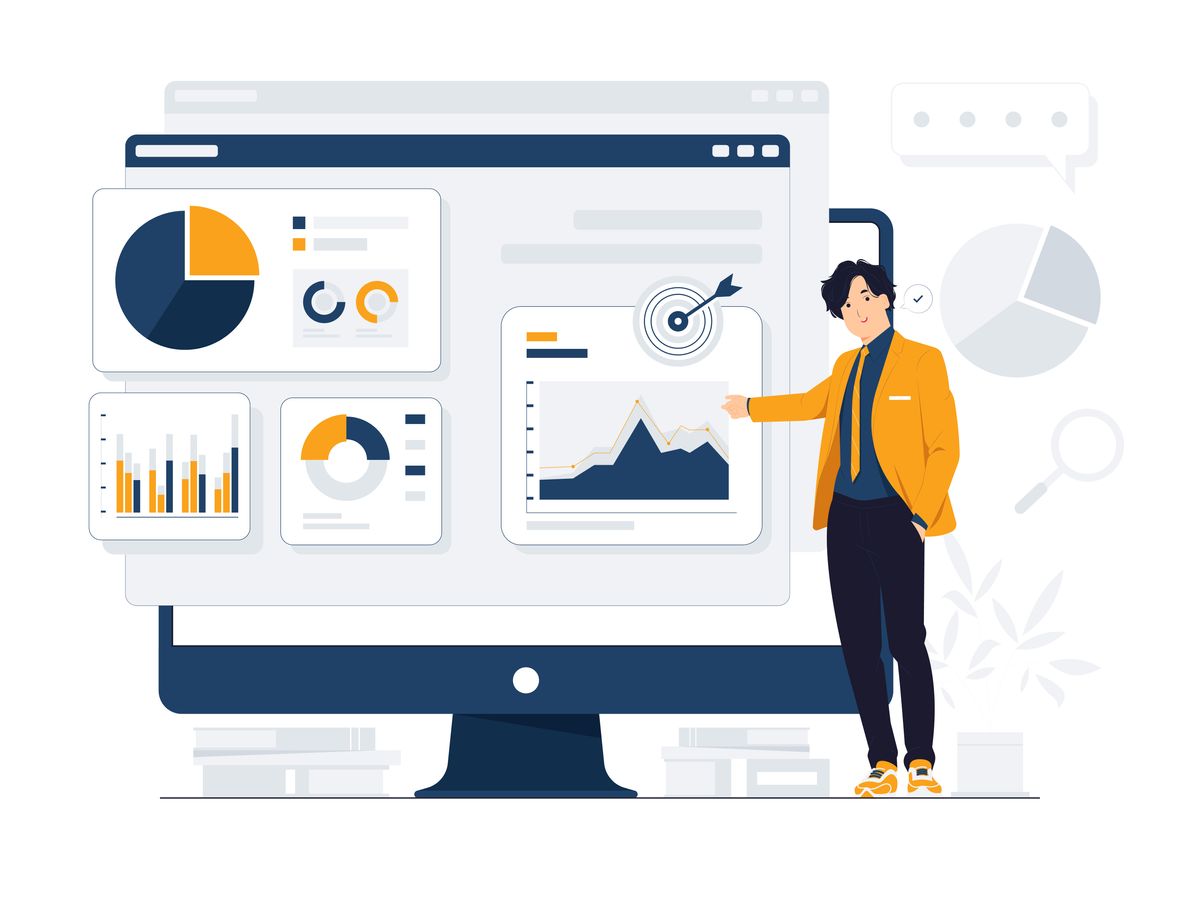
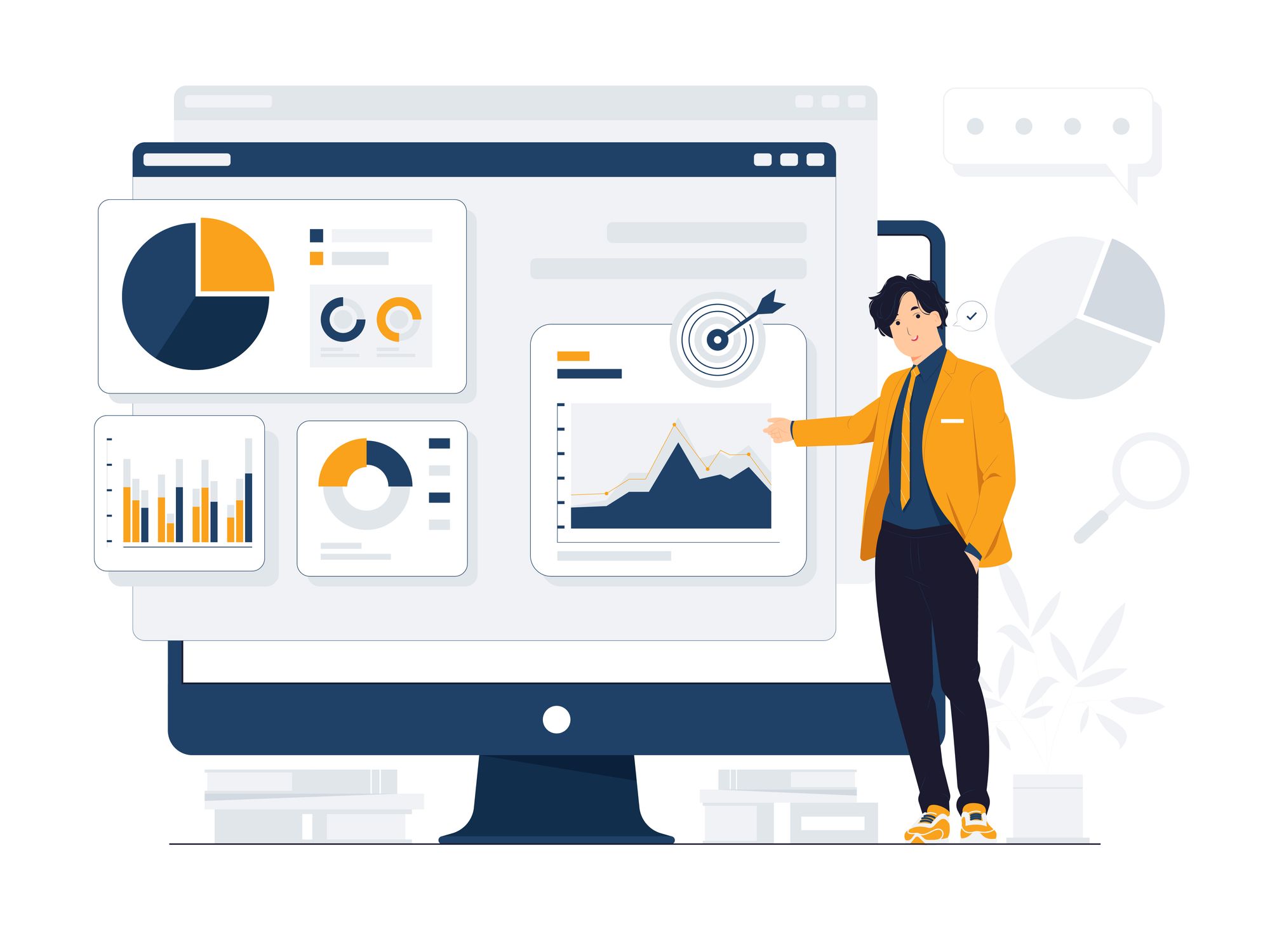
Transform Raw Data into Strategic Assets: The Future of AI-Driven Business Analysis
In the contemporary business landscape, data analysis is no longer a luxury but a necessity for making informed, strategic decisions. While traditional data analysis methods have their merits, they often fall short when it comes to efficiency, scalability, and accuracy. Enter AI and machine learning technologies, which have revolutionized the way businesses approach data analysis. This comprehensive guide aims to equip you with the knowledge to implement AI-powered automated data analysis effectively, thereby amplifying your competitive edge.
Understanding the Fundamentals of Automated Data Analysis Systems
Automated data analysis systems utilize artificial intelligence and machine learning algorithms to sift through voluminous data sets, providing valuable insights that drive business decisions.
Underlying Principles:
- Statistical Analysis: These algorithms scrutinize data to identify relevant patterns, correlations, and trends, thereby aiding in predictive modeling and hypothesis testing.
- Natural Language Processing (NLP): This is pivotal for analyzing unstructured data, such as text documents or social media content, extracting sentiments, and performing topic modeling.
- Anomaly Detection: Particularly useful in sectors like fraud detection and network security, these algorithms identify outliers and irregularities within data sets.
A Step-By-Step Guide to Implementing Automated Data Analysis
Step 1: Data Preparation
The foundation of any successful data analysis initiative lies in the quality of the data used.
- Data Collection: Source data that is accurate, complete, and congruent with the business problem you aim to solve.
- Data Cleaning: This involves removing duplicates, handling missing values, and rectifying inconsistencies.
- Data Quality: Implement stringent data quality checks to ensure the reliability of your analysis.
Step 2: Selecting the Right Tools
The market offers a plethora of AI-driven data analysis tools, each with distinct features and capabilities.
- Scalability: Ensure the tool can handle your data volume and is capable of scaling as your business grows.
- Ease of Use: An intuitive interface and user-friendly workflow are essential for quick adoption.
- Compatibility: The tool must integrate seamlessly with your existing data systems.
Popular Choices:
- Tableau: Renowned for its data visualization capabilities, Tableau offers a user-friendly interface.
- RapidMiner: An end-to-end data science platform with extensive data analysis and machine learning functionalities.
- Google Cloud AutoML: Ideal for those without a coding background, it offers an easy-to-use interface for custom machine learning models.
Step 3: Model Training
Training the model effectively is critical for achieving high predictive accuracy.
- Labeled Data: A labeled dataset serves as the training ground for your chosen machine learning algorithm.
- Model Selection: Choose an algorithm that aligns with your specific requirements and data characteristics.
- Model Optimization: Fine-tune the model by adjusting hyperparameters and evaluating performance through validation data.
Step 4: Real-Time Data Analysis
Implementing real-time analytics can provide actionable insights instantaneously.
- Data Streaming: Utilize technologies like Apache Kafka or Amazon Kinesis to set up a data streaming pipeline.
- Real-Time Analysis: Configure your system to analyze incoming data in real-time, thereby enabling timely decision-making.
- Monitoring and Adjustment: Continually monitor system performance and make necessary adjustments to maintain accuracy and efficiency.
Business Applications and Benefits
Applications:
- Marketing Optimization: Enhance marketing strategies by analyzing consumer behavior and preferences.
- Fraud Detection: Real-time analysis can identify fraudulent activities, thereby safeguarding financial assets.
- Predictive Maintenance: Early detection of equipment failure patterns can lead to proactive maintenance and reduced downtime.
Benefits:
- Increased Efficiency: Automated systems process data with greater speed and less manual intervention.
- Improved Accuracy: AI algorithms significantly reduce human error, offering more reliable insights.
- Actionable Insights: Transform raw data into strategic assets that can drive business growth.
Frequently Asked Questions (FAQs)
What Are the Key Advantages of Automated Data Analysis Over Traditional Methods?
Automated data analysis, powered by AI and machine learning, offers several advantages over traditional manual methods, including:
- Speed: Automated analysis can process large datasets in a fraction of the time.
- Scalability: Easily adaptable to growing data volumes and complexity.
- Accuracy: Minimized human error leads to more reliable insights.
- Real-time Analysis: Capability for real-time decision-making based on incoming data.
Is Specialized Technical Knowledge Required to Implement Automated Data Analysis?
While having a foundational understanding of data science and machine learning can be beneficial, many modern tools are designed with user-friendliness in mind. Platforms like Google Cloud AutoML allow for custom machine learning model creation without extensive coding knowledge.
How Can I Ensure the Quality of Data Used for Analysis?
Data quality is a critical factor in any analysis. Ensure that the data is accurate, complete, and relevant to the problem you're aiming to solve. Data cleaning methods, such as removing duplicates and handling missing values, are essential steps in preparing your data. Additionally, implement quality checks during the data collection process.
Can Automated Data Analysis Systems Be Integrated with Existing Business Infrastructure?
Yes, most modern automated data analysis tools offer robust integration capabilities. It's crucial to ensure that the tool you choose is compatible with your existing data systems for a seamless data flow and analysis process.
What Are Some Common Applications of Automated Data Analysis Across Industries?
Automated data analysis finds applications in a multitude of sectors, including:
- Marketing: For customer segmentation, behavior analysis, and campaign effectiveness.
- Finance: In fraud detection and risk assessment.
- Healthcare: For predictive analytics in patient outcomes.
- Manufacturing: In predictive maintenance and quality control.
By understanding the specific needs and constraints of your industry, you can better leverage automated data analysis for optimal results.
Conclusion
AI-powered automated data analysis is not merely a technological upgrade; it is a strategic enabler that can redefine how businesses operate and compete. By following the guidelines outlined in this article, you can adeptly implement automated data analysis, thereby maximizing efficiency, improving accuracy, and unlocking actionable insights. In a rapidly evolving business landscape, staying ahead of technological advancements is not just beneficial—it is imperative for sustained success.
We encourage you to take the next step in data analysis innovation and explore the myriad opportunities that AI and machine learning technologies present.